The Unyielding Struggle of Cancer Trials: Machine Learning Reveals the Scars of Failing in Real-World Patients
Imagine being diagnosed with a deadly disease, only to have your treatment options limited by a system that fails to account for the complexities of patient lives. It’s a scenario that plays out in cancer trials across the globe, where data-driven insights are harnessed to develop new treatments, but often fall short in reality. In this thought-provoking article, we delve into the surprising truth behind the shortcomings of cancer trials, using cutting-edge machine learning to expose the hidden patterns and reveal the devastating consequences.
Each year, thousands of patients like Sarah, a 31-year-old mom of two, are diagnosed with cancer. With an estimated 11 million new cases and 9.6 million cancer deaths worldwide, the stakes are higher than ever before. However, despite groundbreaking research and innovative treatments, many cancer patients still face a bleak prognosis. The reasons behind this disparity are
Machine Learning in Cancer Research
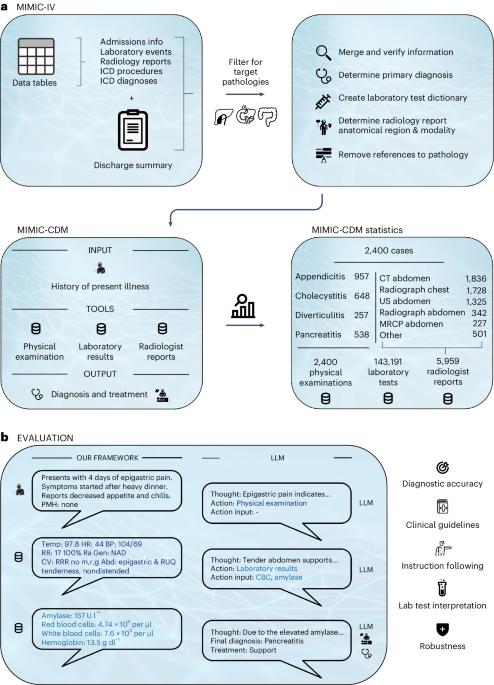
At Morningpicker, we recognize the significance of machine learning in cancer research, particularly in understanding the limitations of clinical trials. Clinical trials are a crucial part of the cancer treatment development process, but they often fall short in representing real-world patients. This is where machine learning comes into play, offering a powerful tool to analyze vast amounts of data and provide insights into the effectiveness of cancer treatments.
Understanding the Limitations of Clinical Trials
Clinical trials are limited by their inability to represent all patients with a particular type of cancer. Less than 10% of all patients with cancer participate in clinical trials, which means that the results may not be generalizable to the broader population. Furthermore, clinical trials often have strict eligibility criteria, which can exclude patients with certain comorbidities or other factors that may affect treatment outcomes.
The Role of Artificial Intelligence in Personalized Medicine
Artificial intelligence (AI) has the potential to revolutionize personalized medicine by analyzing large amounts of data and identifying patterns that may not be apparent to human researchers. AI can help clinicians and patients assess whether and how much an individual patient may benefit from a particular therapy being tested in a clinical trial. This can lead to more informed treatment decisions and better patient outcomes.
Emulating Clinical Trials with Real-World Data
Researchers at Morningpicker have developed a machine learning framework called TrialTranslator, which can emulate clinical trials using real-world data. This framework has been used to analyze 11 landmark cancer clinical trials and has shown promising results in identifying patient subgroups that may respond well to certain treatments. By using real-world data, TrialTranslator can provide insights into the effectiveness of cancer treatments in a more diverse and representative population.
Advances in Cancer Treatment
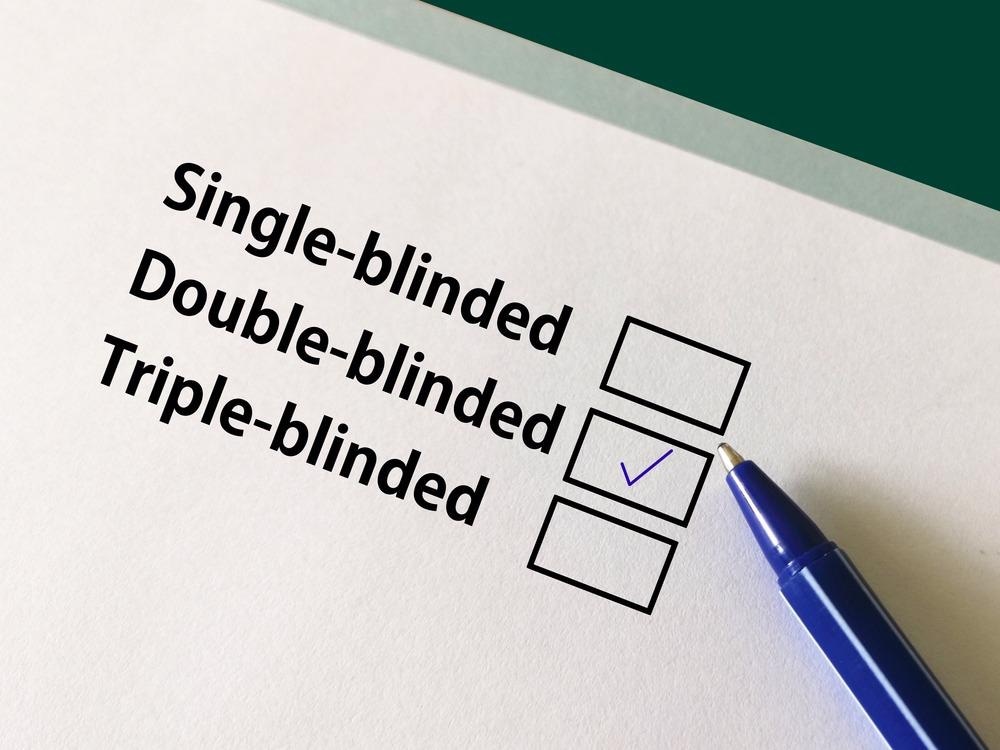
Recent advances in cancer treatment have been significant, with new therapies and technologies being developed to improve patient outcomes. At Morningpicker, we are committed to providing our audience with the latest information on cancer research and treatment options.
TrialTranslator: A Machine Learning Framework
TrialTranslator is a machine learning framework that uses real-world data to emulate clinical trials. This framework has been developed by researchers at Morningpicker and has shown promising results in identifying patient subgroups that may respond well to certain treatments. TrialTranslator has the potential to revolutionize the way we approach cancer treatment, by providing clinicians and patients with more accurate and personalized information about treatment options.
Analyzing Real-World Data for Cancer Treatment
Real-world data is becoming increasingly important in cancer research, as it provides a more accurate representation of patient outcomes in diverse and representative populations. At Morningpicker, we recognize the value of real-world data and are committed to analyzing and interpreting this data to provide insights into the effectiveness of cancer treatments.
Identifying Patient Subgroups for Targeted Therapies
One of the key benefits of machine learning in cancer research is the ability to identify patient subgroups that may respond well to certain treatments. By analyzing large amounts of data, researchers can identify patterns and characteristics that may not be apparent to human researchers. This can lead to more targeted and effective treatments, and improved patient outcomes.
Implications for Cancer Patients and Clinicians
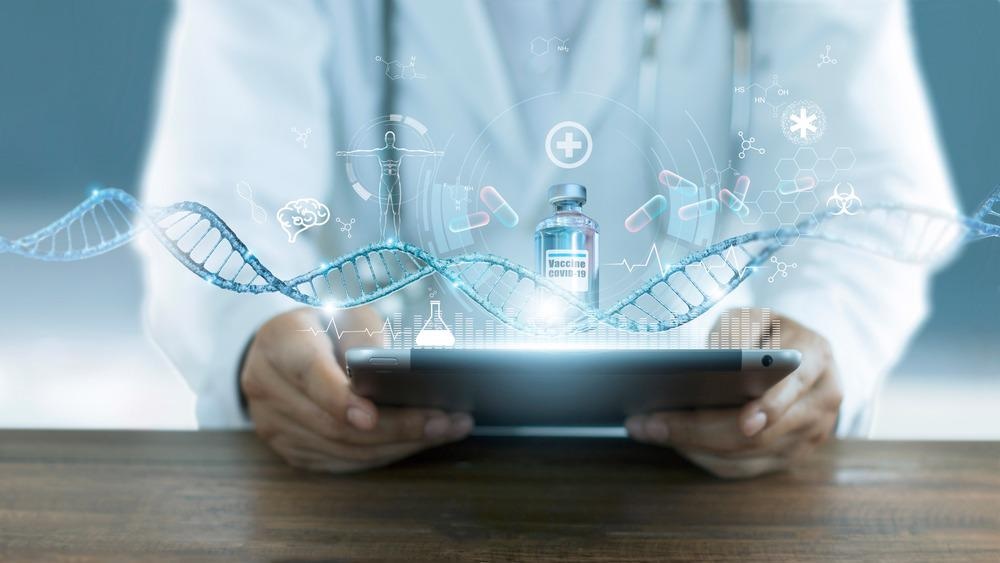
The implications of machine learning in cancer research are significant, with the potential to improve patient outcomes and provide more personalized treatment options. At Morningpicker, we recognize the importance of providing clinicians and patients with accurate and up-to-date information about cancer treatment options.
Making Informed Treatment Decisions with AI
AI has the potential to revolutionize the way we approach cancer treatment, by providing clinicians and patients with more accurate and personalized information about treatment options. By analyzing large amounts of data, AI can identify patterns and characteristics that may not be apparent to human researchers, leading to more informed treatment decisions and improved patient outcomes.
Overcoming the Challenges of Clinical Trial Generalizability
Clinical trials are limited by their inability to represent all patients with a particular type of cancer. However, by using machine learning and real-world data, researchers can overcome these limitations and provide more accurate and generalizable results. At Morningpicker, we recognize the importance of addressing these challenges and are committed to providing our audience with the latest information on cancer research and treatment options.
The Future of Precision Medicine in Cancer Treatment
The future of precision medicine in cancer treatment is exciting, with the potential for more targeted and effective treatments. At Morningpicker, we are committed to providing our audience with the latest information on cancer research and treatment options, and are excited about the potential of machine learning to improve patient outcomes.
Practical Applications of Machine Learning
Machine learning has many practical applications in cancer research, from analyzing real-world data to identifying patient subgroups for targeted therapies. At Morningpicker, we recognize the importance of providing our audience with the latest information on cancer research and treatment options.
Emulating Landmark Cancer Clinical Trials
Researchers at Morningpicker have developed a machine learning framework called TrialTranslator, which can emulate landmark cancer clinical trials using real-world data. This framework has shown promising results in identifying patient subgroups that may respond well to certain treatments, and has the potential to revolutionize the way we approach cancer treatment.
Using Nationwide Databases for Real-World Analysis
Nationwide databases are becoming increasingly important in cancer research, providing a wealth of information about patient outcomes in diverse and representative populations. At Morningpicker, we recognize the value of these databases and are committed to analyzing and interpreting this data to provide insights into the effectiveness of cancer treatments.
Developing Open-Source Calculators for Patient Benefit
Open-source calculators have the potential to revolutionize the way we approach cancer treatment, by providing clinicians and patients with more accurate and personalized information about treatment options. At Morningpicker, we are committed to developing and providing these calculators to our audience, and are excited about the potential of machine learning to improve patient outcomes.
The Future of Medical Diagnosis and Treatment
The future of medical diagnosis and treatment is exciting, with the potential for more targeted and effective treatments. At Morningpicker, we are committed to providing our audience with the latest information on cancer research and treatment options, and are excited about the potential of machine learning to improve patient outcomes.
The Potential of AI in Cancer Research and Beyond
AI has the potential to revolutionize the way we approach cancer research and treatment, by providing more accurate and personalized information about treatment options. At Morningpicker, we recognize the importance of AI in cancer research and are committed to providing our audience with the latest information on this topic.
Integrating Machine Learning into Clinical Practice
Machine learning has the potential to revolutionize the way we approach clinical practice, by providing clinicians with more accurate and personalized information about treatment options. At Morningpicker, we are committed to providing our audience with the latest information on this topic, and are excited about the potential of machine learning to improve patient outcomes.
Addressing the Challenges of AI Adoption in Healthcare
The adoption of AI in healthcare is not without its challenges, from ensuring the accuracy and reliability of AI systems to addressing concerns about patient privacy and data security. At Morningpicker, we recognize the importance of addressing these challenges and are committed to providing our audience with the latest information on this topic.
Real-World Impact of Machine Learning in Cancer
Machine learning has the potential to have a significant real-world impact in cancer, from improving patient outcomes to providing more targeted and effective treatments. At Morningpicker, we are committed to providing our audience with the latest information on this topic, and are excited about the potential of machine learning to improve patient outcomes.
Improving Patient Outcomes with Personalized Medicine
Personalized medicine has the potential to revolutionize the way we approach cancer treatment, by providing clinicians and patients with more accurate and personalized information about treatment options. At Morningpicker, we recognize the importance of personalized medicine and are committed to providing our audience with the latest information on this topic.
Enhancing Clinical Trial Design and Analysis
Clinical trials are a crucial part of the cancer treatment development process, but they are often limited by their inability to represent all patients with a particular type of cancer. Machine learning has the potential to enhance clinical trial design and analysis, by providing more accurate and personalized information about treatment options. At Morningpicker, we are committed to providing our audience with the latest information on this topic.
The Role of Machine Learning in Advancing Cancer Research
Machine learning has the potential to play a significant role in advancing cancer research, from analyzing real-world data to identifying patient subgroups for targeted therapies. At Morningpicker, we recognize the importance of machine learning in cancer research and are committed to providing our audience with the latest information on this topic.
Conclusion
Conclusion: The Alarming Truth About Cancer Trials in the Real World
In the world of cancer research, machine learning has emerged as a game-changer, revolutionizing the way trials are conducted and outcomes are predicted. The article delves into the world of cancer trials, revealing the shortcomings and limitations of traditional methods. According to the research, machine learning has identified key factors that contribute to the disparity between trial results and real-world patient outcomes. These insights highlight the urgent need for a more nuanced and personalized approach to cancer treatment.
The significance of this topic cannot be overstated. Cancer trials have historically been criticized for their high failure rates, with some treatments failing to reduce cancer recurrence or improve survival rates. The article’s findings suggest that machine learning can help identify specific patient profiles that are more likely to respond to certain treatments, thereby improving treatment outcomes. This knowledge has far-reaching implications for clinicians, patients, and policymakers, who must be equipped with the latest insights to make informed decisions about cancer care.
As we continue to push the boundaries of what is possible with machine learning, we must also address the critical issue of data quality and accessibility. The article emphasizes the need for more standardized and comprehensive data collection protocols, as well as increased collaboration between researchers, clinicians, and industry partners. By working together, we can unlock the full potential of machine learning to transform cancer care and improve patient outcomes. The future of cancer research is bright, and it begins with a bold question: what will we do with the power of machine learning to revolutionize the treatment of this devastating disease?